Unlocking the Potential of Meta AI: Exploring Applications, Techniques, Challenges, and Ethics
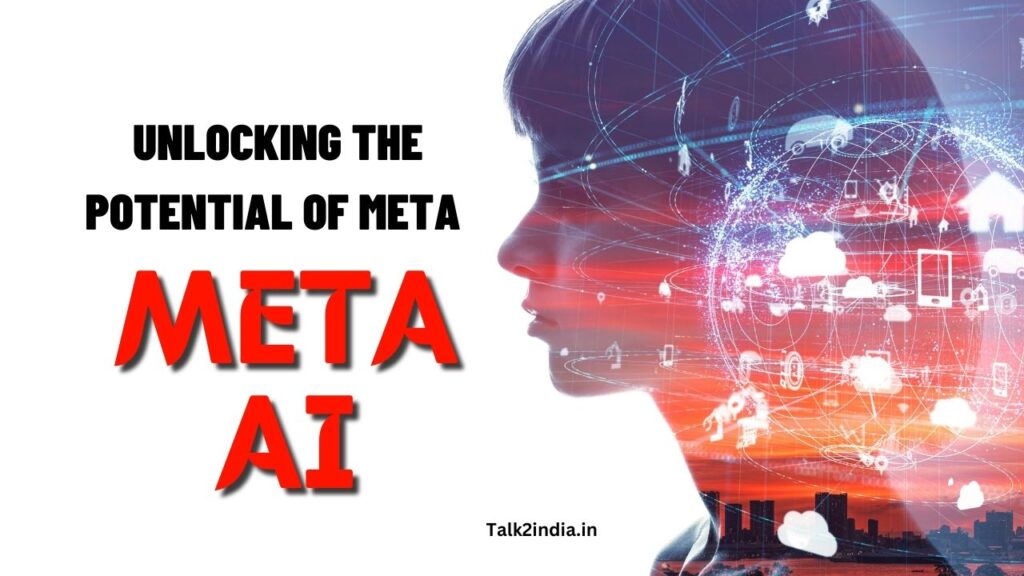
Artificial intelligence (AI) has come a long way since its inception, and its potential continues to grow with the advent of meta-AI. Meta AI refers to the application of AI techniques to enhance the performance and capabilities of other AI systems. By leveraging transfer learning, meta-learning, and other techniques, Meta AI is poised to revolutionize the field of AI and enable more efficient and effective decision-making across various domains.
Also Read: The Ultimate Guide to Make Money with ChatGPT
What is “Meta AI”?
Meta AI is an umbrella term that encompasses various approaches to improving the performance of AI systems. At its core, Meta AI is about learning to learn. This means that an AI system can improve its own performance by learning from its own experiences as well as from the experiences of other AI systems. Meta AI can be used to enhance the capabilities of existing AI systems or to create entirely new ones.
Meta AI Applications
Meta AI has numerous applications across various domains. Here are a few examples:
- Healthcare: Meta AI can be used to optimize clinical decision-making by analyzing patient data and identifying patterns that may not be apparent to human physicians. This can improve patient outcomes and reduce costs.
- Finance: Meta AI can be used to improve financial forecasting by analyzing market trends and identifying patterns that may not be apparent to human analysts. This can help financial institutions make better investment decisions and reduce risk.
- Transportation: Meta AI can be used to enhance autonomous driving by analyzing real-time traffic data and identifying patterns that may not be apparent to human drivers. This can improve safety and reduce congestion on the roads.
- Education: Meta AI can be used to personalize educational experiences by analyzing student data and identifying patterns that may not be apparent to human teachers. This can improve student engagement and academic performance.
Techniques used in Meta AI
There are various techniques used in Meta AI, including Bayesian optimization, reinforcement learning, and evolutionary algorithms. Here’s a brief overview of each technique:
- Bayesian optimization: Bayesian optimization is a technique that can be used to optimize the hyperparameters of machine learning models. This method uses a probabilistic model to estimate how well different configurations of hyperparameters will work and then chooses the best configuration based on how well it is expected to work.
- Reinforcement learning: Reinforcement learning is a technique that can be used to teach an AI system to learn by trial and error. In this method, the AI system learns by getting rewards or punishments based on what it does.
- Evolutionary algorithms: Evolutionary algorithms are a family of optimization algorithms that mimic the process of natural selection. In this method, a population of solutions is changed over many generations, with the best solutions surviving and reproducing to make the next generation of solutions.
Also Read: 5 Amazing Chat GPT Features For Seamless Interactions
Challenges in Meta-AI
While Meta AI has immense potential, it also faces several challenges. Here are a few examples:
- Developing effective transfer learning algorithms: Transfer learning is a technique that involves transferring knowledge from one AI system to another. But it can be hard to make transfer learning algorithms that work well because the information from one AI system may not always be useful for another.
- Handling uncertainty and incomplete data: In real-world scenarios, data may be uncertain or incomplete, which can make it challenging for AI systems to learn effectively. Meta AI needs to develop techniques to handle such data effectively.
- Mitigating the risk of catastrophic failure: In highly complex and dynamic systems, catastrophic failure can be a significant risk. Meta AI needs to come up with ways to reduce these risks and keep the systems it improves safety.
Conclusion
Meta AI has the potential to revolutionize the field of artificial intelligence by enabling more efficient and effective decision-making across various domains. It leverages transfer learning, meta-learning, and other techniques to
enable AI systems to learn and adapt to new tasks and environments more quickly and effectively. Meta AI is likely to have a big impact on the world because it can be used in healthcare, finance, transportation, education, and many other fields.
However, as with any new field, Meta AI faces a number of challenges. Developing effective transfer learning algorithms, handling uncertainty and incomplete data, and mitigating the risk of catastrophic failure are just a few of the challenges that need to be addressed. However, as researchers and practitioners continue to push the boundaries of what is possible with meta AI, we can expect many exciting developments in the coming years.
As meta-AI continues to evolve, it will be essential to consider the ethical implications of its use. While meta-AI has the potential to improve decision-making and increase efficiency, it also has the potential to amplify biases and exacerbate inequalities. It will be important to ensure that Meta AI is developed and used in a way that is fair, transparent, and accountable.
In conclusion, Meta AI represents a significant advance in the field of artificial intelligence. With its potential to enhance the performance of existing AI systems and create entirely new ones, Meta AI has the potential to revolutionize a wide range of domains. However, as with any emerging technology, it will be essential to address the challenges and ethical considerations associated with its use. By doing so, we can unlock the full potential of Meta AI and create a brighter future for all.