Understanding Generative AI: Goals, Key Features, and Challenges
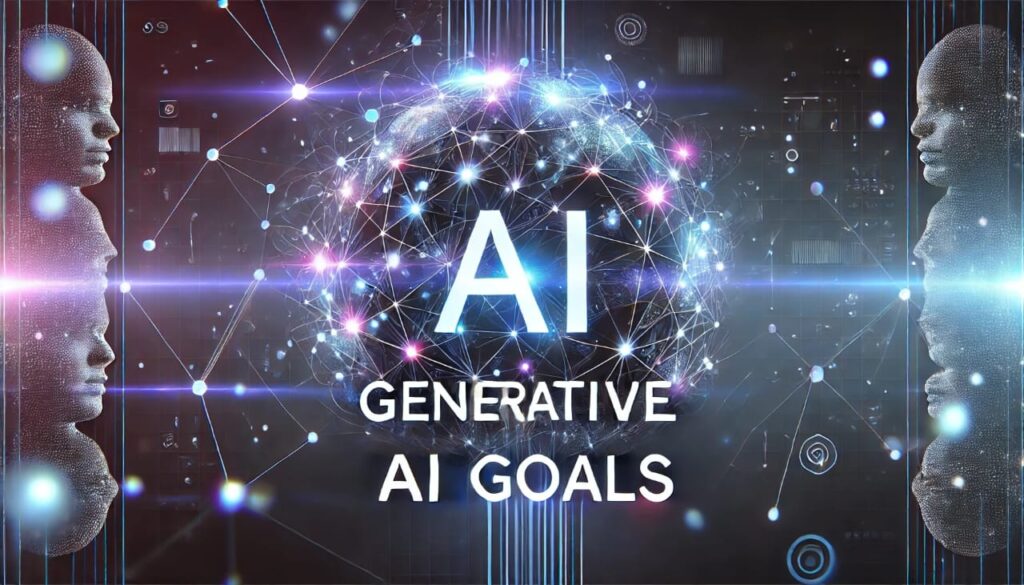
Generative AI is transforming various industries, from content creation to healthcare. By simulating realistic data, generative AI models open up new possibilities in fields that rely on creativity, automation, and complex data analysis. In this blog, we’ll explore the main goals, key features, and data-related challenges of generative AI, as well as its foundational elements and future potential.
What is Generative AI?
Generative AI is a branch of artificial intelligence that creates new data by learning from existing data patterns. Unlike traditional AI models, which mostly focus on data analysis and pattern recognition, generative AI can produce original, realistic outputs such as images, text, and even music. This ability to generate rather than simply interpret data makes generative AI a powerful tool for industries that require innovation and data synthesis, from digital media to scientific research.
At its core, generative AI works by using machine learning techniques like neural networks to process large datasets and identify complex patterns. One of the most popular types of generative AI models is the Generative Adversarial Network (GAN), where two networks compete to create increasingly realistic outputs. This process results in high-quality, authentic-looking data that can be used in applications like synthetic media, product designs, and interactive chatbots.
What is the Main Goal of Generative AI?
The primary goal of generative AI is to create new data that closely resembles the data it was trained on. By doing this, generative AI opens up avenues for advanced applications in a variety of fields:
- Media and Entertainment: Generative AI is widely used to generate realistic images and videos, design game assets, and even compose music. By producing high-quality synthetic media, generative AI can reduce production costs and streamline creative processes.
- Healthcare: Generative AI models can create realistic medical images to aid in diagnosis and research, particularly in cases where real patient data is limited. They can also simulate chemical compounds for drug discovery, potentially accelerating medical breakthroughs.
- Customer Service: Through chatbots and virtual assistants, generative AI can provide more natural, responsive interactions with users, allowing for better customer experiences and personalized recommendations.
Generative AI’s main goal is to improve the capabilities of various industries by providing data-rich resources that enhance creativity, reduce human effort, and facilitate faster decision-making.
Key Features of Generative AI
Generative AI offers unique features that set it apart from other AI technologies. Below are some of the defining characteristics of generative AI:
Data Generation
One of the key features of generative AI is its ability to create new data from a small sample. For instance, a generative AI model trained on a dataset of images can produce new images that look like the originals but are entirely new. This feature is useful in fields where there is limited original data, like rare medical conditions.
Pattern Recognition
Generative AI models excel in identifying complex patterns within datasets. By recognizing intricate relationships, these models can generate data that is not only similar to the training data but also tailored to specific purposes. This feature is valuable for applications like predictive text, automated content creation, and personalized product recommendations.
Flexibility and Scalability
Generative AI models are highly adaptable, making them suitable for various applications across different industries. For instance, a single model can be adapted to generate different types of content or images with slight modifications, allowing for a scalable solution that can grow with the demands of the organization.
By incorporating these features, generative AI becomes an invaluable tool for industries seeking innovation, efficiency, and productivity.
What Are Some of the Key Elements of AI?
Generative AI, like all AI models, relies on several foundational elements to operate effectively. Below are some of the key elements that contribute to its success:
- Algorithms: Generative AI uses complex algorithms, including deep learning models and neural networks, to process data and produce new information. These algorithms are essential for generating high-quality, realistic outputs.
- Data Processing: High-quality data is crucial for generative AI models. The data must be preprocessed and cleaned to eliminate noise, bias, and inconsistencies, allowing the AI to learn from accurate information.
- Machine Learning: Generative AI relies on machine learning to train on data. Through techniques like supervised and unsupervised learning, these models can make accurate predictions and generate realistic outputs.
- Neural Networks: Neural networks, especially GANs, are foundational for generative AI. They use a multi-layered structure that allows them to process complex data patterns, enabling the creation of high-quality synthetic data.
These elements form the backbone of AI systems and provide the structural support needed for generative AI to function.
Challenges Generative AI Faces with Data
While generative AI offers remarkable capabilities, it also faces significant challenges, especially with respect to data. Some of these key challenges are discussed below:
Data Quality and Quantity
For generative AI models to function effectively, they require high-quality and extensive datasets. However, in certain fields, obtaining such datasets can be challenging due to privacy concerns, limited availability, or the cost of data collection. Inadequate data quality or quantity can lead to poor model performance and low-quality outputs, reducing the reliability of the AI-generated content.
Bias in Data
Bias in training data is a persistent issue in generative AI. If the data fed into the model contains biases—be it cultural, social, or demographic—the outputs will likely reflect those biases. This can result in skewed or inappropriate outputs, impacting the model’s effectiveness and possibly leading to ethical concerns.
Privacy and Security
Data privacy and security concerns are especially relevant in generative AI applications that handle sensitive information. For instance, using generative AI in healthcare to generate synthetic patient data may pose privacy risks if not properly anonymized. Additionally, there are concerns that malicious actors could misuse generative AI to create false information or deepfake content.
Addressing these challenges is critical for the safe and effective use of generative AI across different applications, ensuring its outputs are ethical, reliable, and secure.
Future Prospects of Generative AI
As generative AI continues to evolve, its applications are expected to expand across various industries. From enhancing user experiences with more realistic virtual environments to creating advanced predictive models in scientific research, the future potential of generative AI is vast. With ongoing improvements in algorithms and data processing capabilities, generative AI could significantly shape the digital landscape, enabling new levels of creativity, automation, and problem-solving.
Conclusion
Generative AI stands out for its ability to create new data, offering immense value across industries that rely on data generation, innovation, and automation. While it presents challenges, particularly around data quality, bias, and privacy, generative AI’s potential for driving progress and enhancing applications is undeniable. By understanding both its strengths and limitations, businesses and individuals can harness generative AI to stay competitive in an increasingly AI-driven world.